Levi's Intelligent Inventory Allocation System
Fast-paced AI design & business transformation
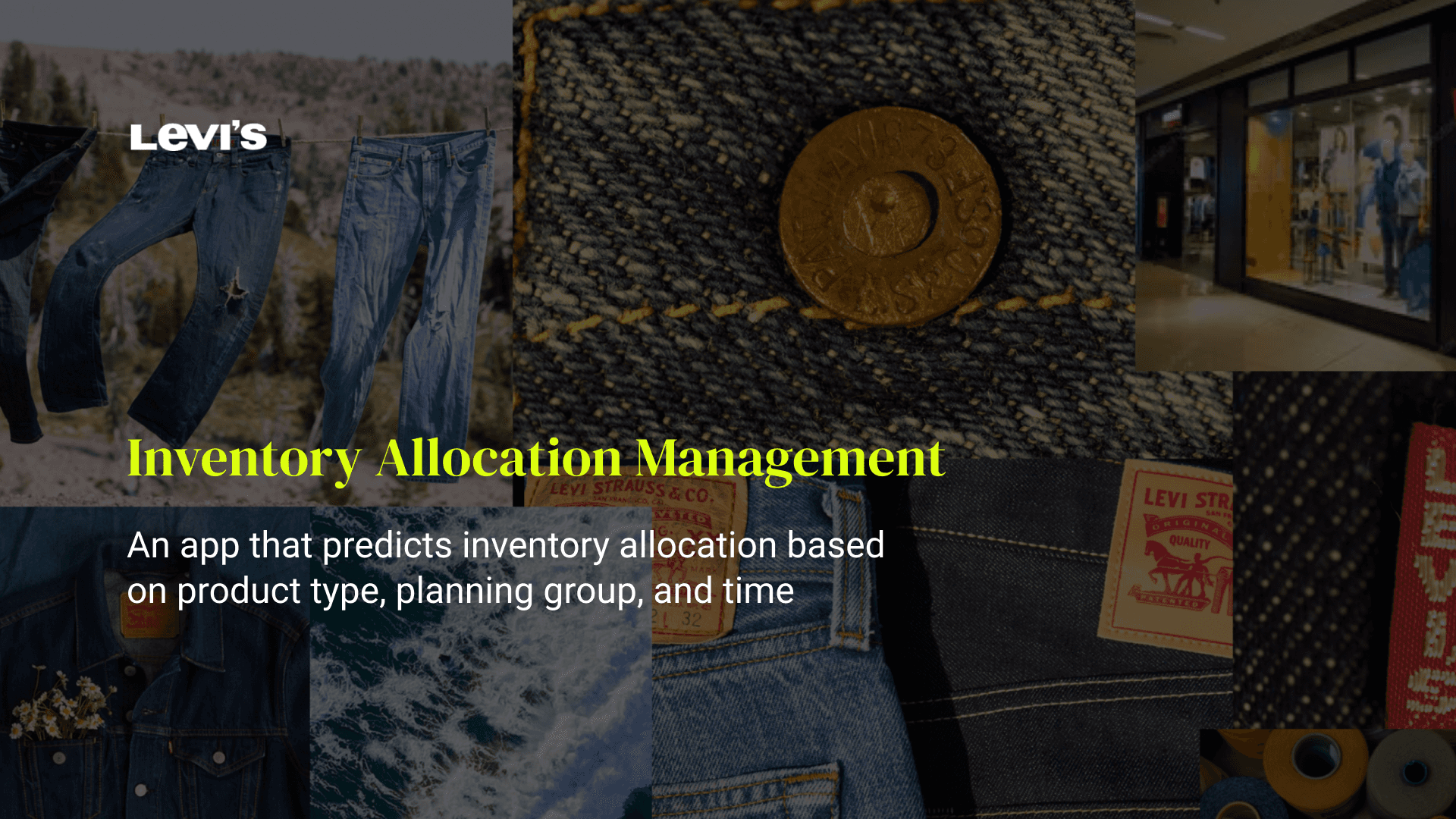
Summary
Inventory management in the apparel industry is a high-stakes challenge, especially during market disruptions like the COVID-19 pandemic. When Levi’s struggled with manual processes, inaccurate demand forecasting, and slow response times, SAP partnered with them to design a machine learning-powered inventory allocation system.
As the customer engagement and design lead, I directed the research, solution design, and prototyping of this system. Over three months, we developed an AI-driven allocation model and an interactive dashboard that improved accuracy by 30.8%, automated tedious manual tasks, and enhanced real-time decision-making for inventory planners.
This case highlights my ability to lead cross-functional teams, design AI-powered enterprise solutions, and translate complex supply chain challenges into scalable digital tools.
Project Overview
Inventory allocation is a critical function in the apparel industry, balancing demand, supply chain fluctuations, and product availability across multiple locations. Before this project, Levi’s relied on manual processes and rigid forecasting models, which were ineffective during the unpredictable market shifts of the COVID-19 pandemic.
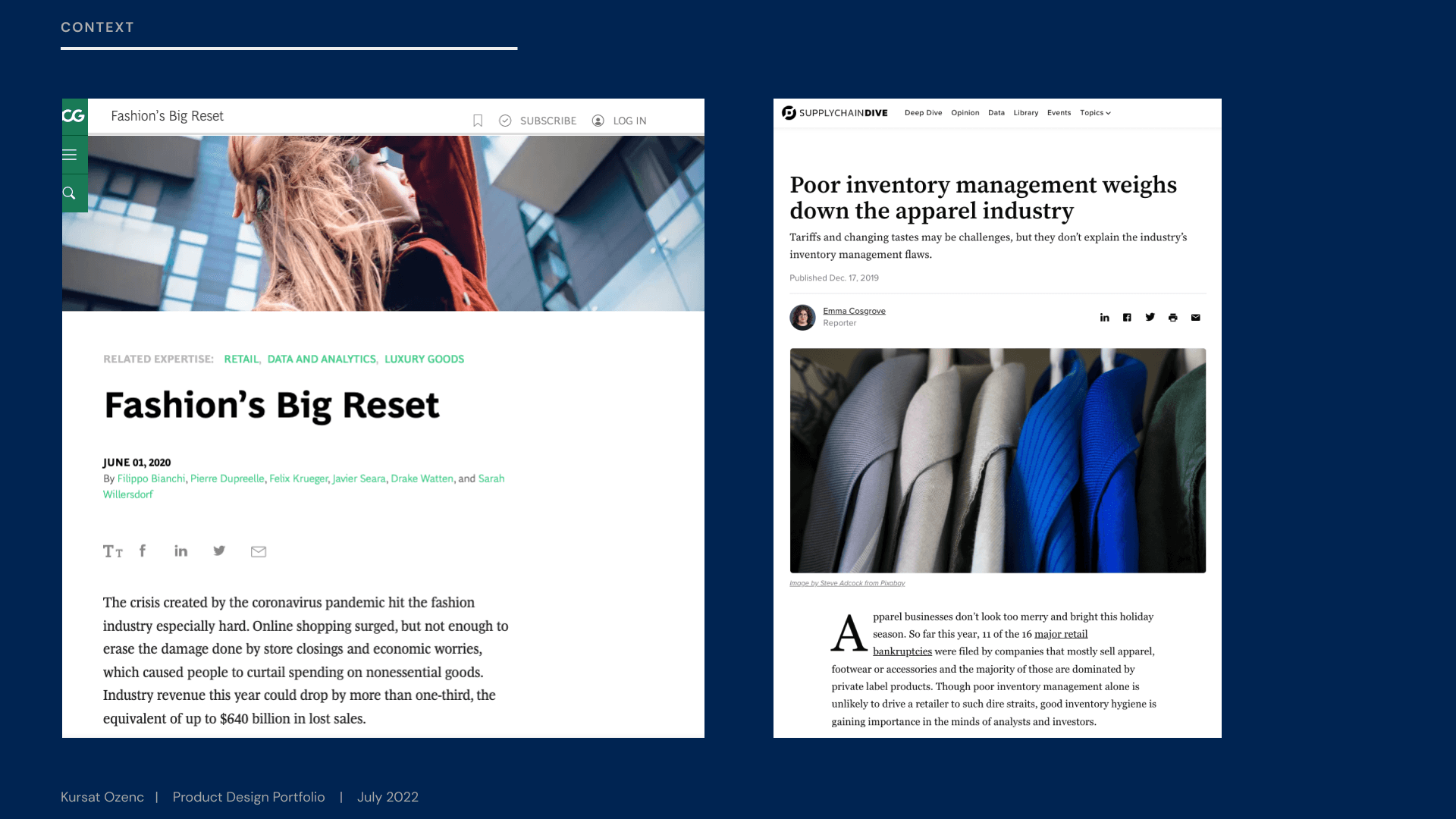
When their SAP-based inventory system failed to adapt, Levi’s and SAP collaborated to create an intelligent inventory allocation platform that would increase accuracy, automate adjustments, and improve operational efficiency.
This project required a user-centered approach to uncover core pain points, improve forecasting with AI, and create a seamless digital experience for inventory planners.
Key Challenges
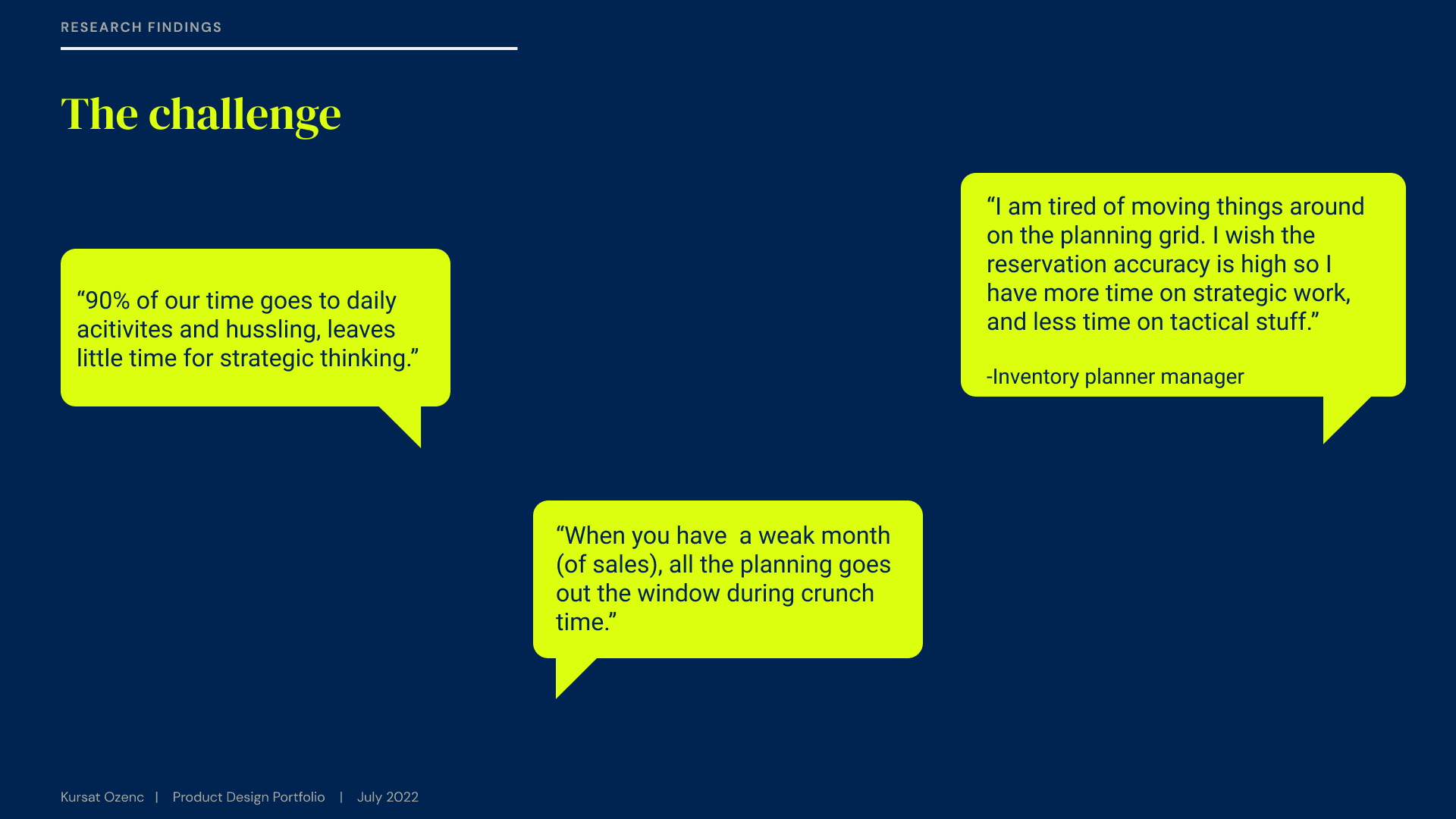
Levi’s faced significant inventory planning inefficiencies that led to both financial losses and operational bottlenecks. The main issues included:
- Inaccurate inventory forecasting – Traditional models failed to adjust to real-world demand fluctuations, leading to overstock and understock situations.
- Inefficient reservation management – Inventory planners struggled with manual stock allocation, making it difficult to respond quickly to supply chain disruptions.
- Overwhelming daily tasks – With constant manual adjustments, planners had little time for strategy, optimization, or proactive decision-making.
- Slow response to disruptions – Without real-time insights, the team often reacted too late to supply chain changes, increasing inefficiencies.
These challenges highlighted the urgent need for a data-driven, automated system that could improve both speed and accuracy in inventory decision-making.
Approach & Solution
To solve these issues, I led the design and development of a machine learning-powered inventory allocation system, ensuring that technology and user needs aligned. Our team delivered a functional prototype in three months by following a structured, iterative process.
Research and Discovery
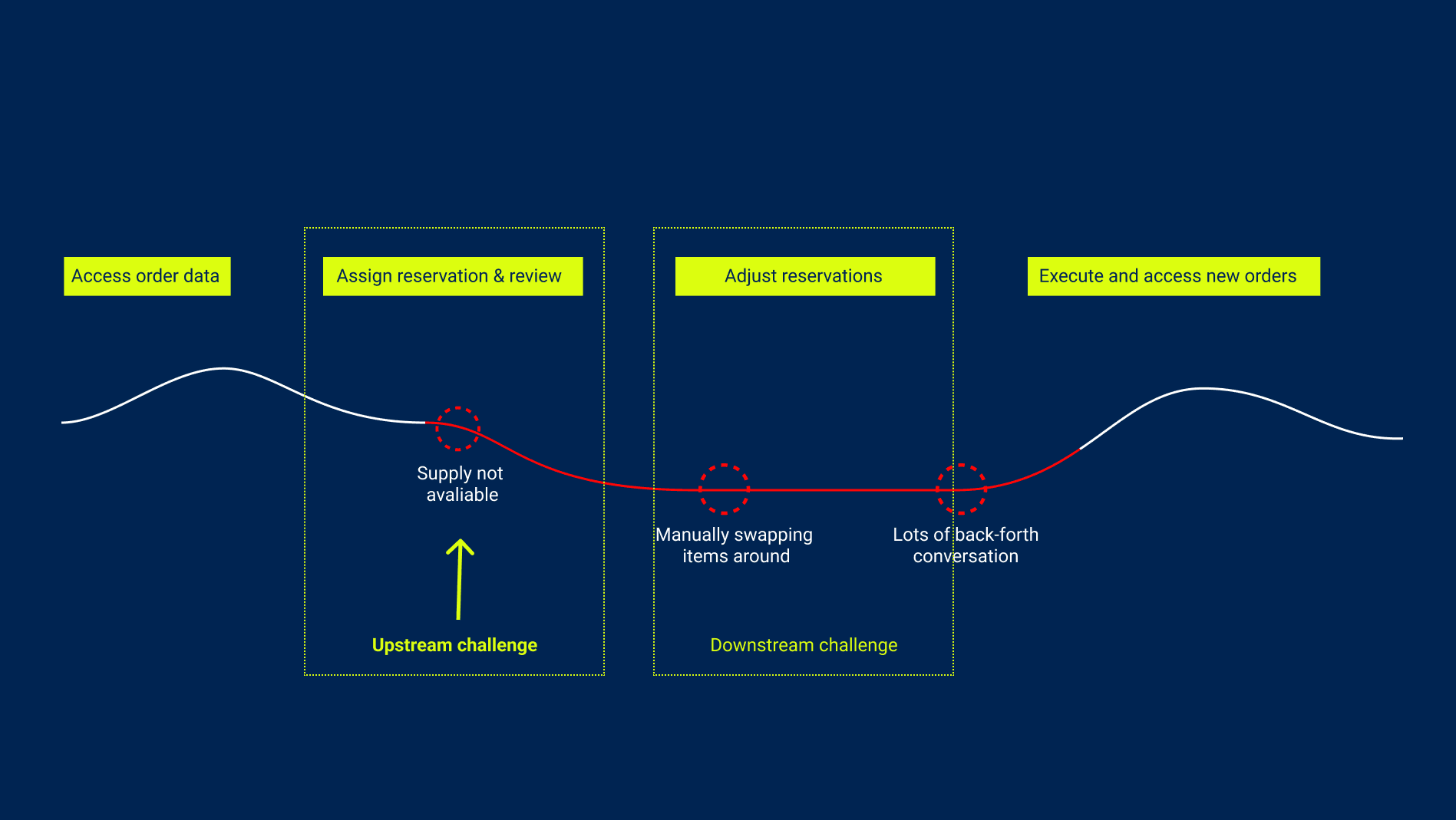
The first step was to gain deep insights into how inventory planners worked, what challenges they faced, and where automation could help.
- Conducted workshops and interviews with Levi’s planners to map existing workflows and pinpoint manual bottlenecks.
- Analyzed historical sales data, supply chain adjustments, and demand forecasting challenges to identify root causes of inaccuracies.
- Mapped current inventory allocation models to determine areas where machine learning could enhance accuracy and efficiency.
Solution Design
With these insights, we designed a machine learning-based system to dynamically adjust inventory allocation based on historical patterns and external factors.
- Built a predictive AI model that learns from past sales data, market trends, and supply chain variables to optimize stock distribution.
- Designed a real-time inventory visualization dashboard, giving planners a clear, interactive view of stock levels and allocation accuracy.
- Integrated seamlessly with Levi’s SAP systems, ensuring compatibility with existing workflows.
Prototyping and Testing
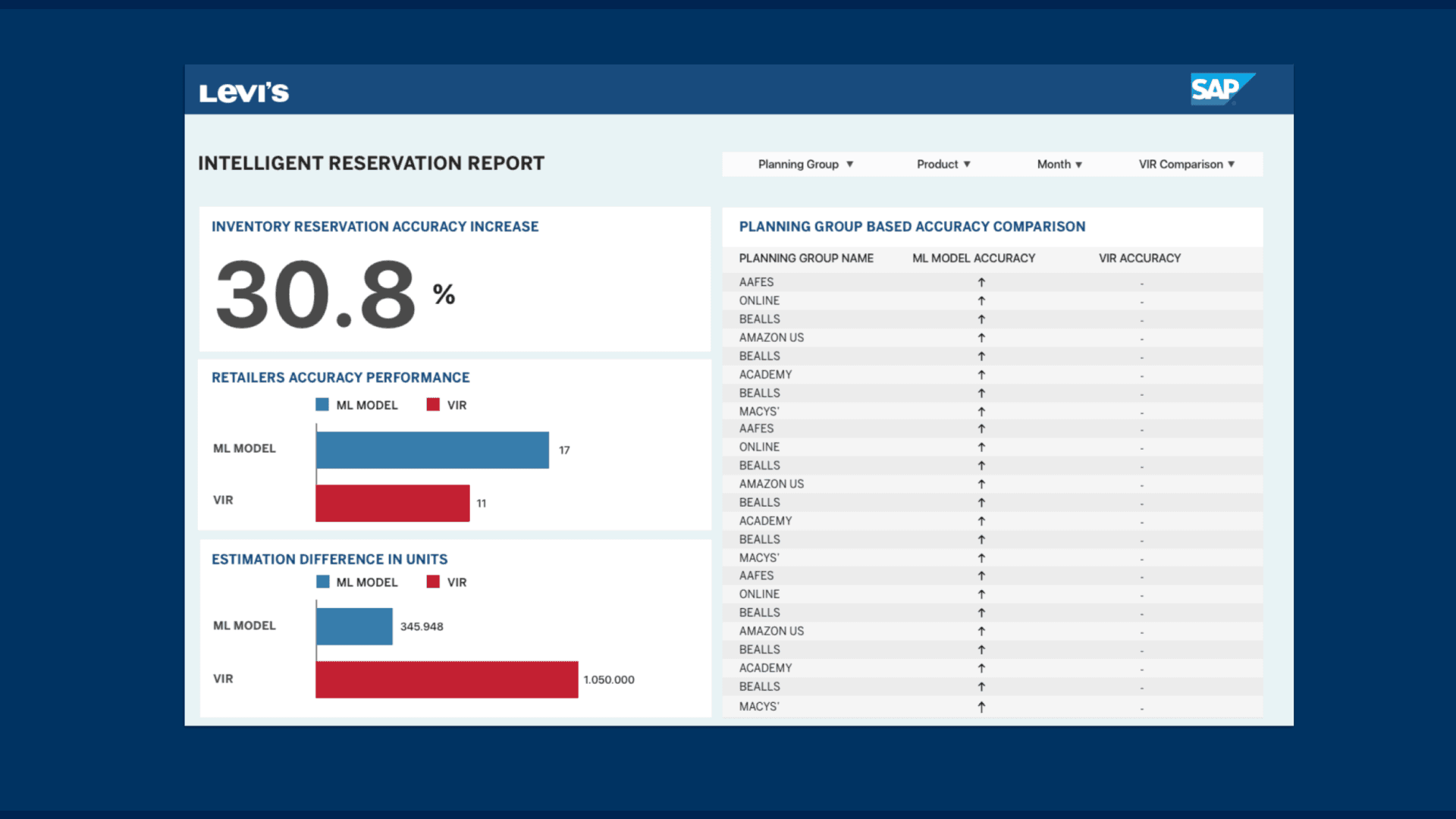
To validate the solution, we built an interactive prototype that demonstrated the impact of machine learning-driven allocation.
- Developed dynamic accuracy metrics to measure improvements in stock allocation over time.
- Created actionable insights and alerts, helping planners make quick, data-driven decisions instead of relying on manual guesswork.
- Streamlined the reservation adjustment process, reducing unnecessary manual intervention while improving agility in supply chain responses.
This process ensured that the final system was both technically feasible and highly usable for Levi’s inventory planners.
Impact & Business Value
The intelligent inventory allocation system delivered measurable improvements in efficiency, accuracy, and strategic decision-making.
- Improved forecasting accuracy by 30.8%, reducing both overstock and understock risks.
- Reduced manual workload, freeing up planners’ time for strategic inventory optimization.
- Accelerated supply chain response times, enabling quicker adjustments to market demand shifts.
- Strengthened SAP’s position as a cloud-driven operational partner, increasing customer confidence in data-driven inventory management.
This solution not only addressed Levi’s immediate supply chain challenges but also provided a scalable foundation for future AI-driven inventory management across the apparel industry.
Key Learnings & Strategic Insights
This project revealed critical insights about the intersection of AI, supply chain management, and user-centered enterprise design.
- AI-powered automation must align with human workflows – The biggest impact came from ensuring that machine learning insights were actionable and interpretable by human planners, rather than replacing their decision-making entirely.
- Real-time visibility is critical in inventory allocation – A static forecasting model isn’t enough; planners need live dashboards that allow them to monitor stock movements and adjust proactively.
- Seamless enterprise integration is key – Since Levi’s relied on existing SAP infrastructure, the solution had to blend into their ecosystem, not require a disruptive overhaul.
- Fast iteration leads to rapid adoption – Delivering a pre-production prototype in three months allowed stakeholders to engage early, ensuring that the final product was user-aligned and ready for deployment.
These learnings reinforce the importance of bridging AI capabilities with real business needs, ensuring that innovation translates into practical, scalable, and user-centered enterprise solutions.
Takeaways
This case study demonstrates my ability to lead AI-driven enterprise innovation, integrate human-centered design with data science, and drive measurable business impact.
- I create enterprise AI solutions that solve real business challenges – This project shows my ability to apply machine learning in a practical, results-driven way.
- I design scalable, user-friendly digital tools – The interactive dashboard and automation features made complex AI outputs usable and actionable for inventory planners.
- I lead fast-paced, high-impact innovation – Delivering a working prototype in three months showcases my ability to rapidly move from insights to execution.